MaxDiff Analysis
MaxDiff (also known as Maximum Difference Scaling or Best–Worst Scaling) is a statistical technique that creates a robust ranking of different items, such as product features. MaxDiff is an alternative to conjoint analysis in which the respondent has to indicate which feature is most important or most desirable, and which is least important or desirable. Conjointly’s novel robust approach to MaxDiff allows for:
- Testing of multiple attributes in the same survey.
- Brand-Specific combinations of attributes for when each brand is substantially different (to enable that, first create a Brand-Specific Conjoint and then convert it into the MaxDiff variety).
- Creating a Feature Placement Matrix to classify features by both importance and willingness to pay (to enable that, include Van Westendorp questions in your MaxDiff experiment).
- Simulation of preference shares at a highly indicative level.
Traditionally, MaxDiff treats each product as an individual item, whilst conjoint treats products as a combination of attributes and levels. As such, the conjoint analysis produces rankings for particular products by summing the preference scores for each attribute level of a product, whilst MaxDiff produces rankings by polling the respondents directly.
Using MaxDiff surveys to determine which colours are the most popular for the customers.
MaxDiff surveys ask the respondents directly about each product as an individual item.
MaxDiff questions can be automatically translated to more than 30 languages.
Bring your own respondents or source real human respondents swiftly and at scale through us.
Main outputs of MaxDiff Analysis
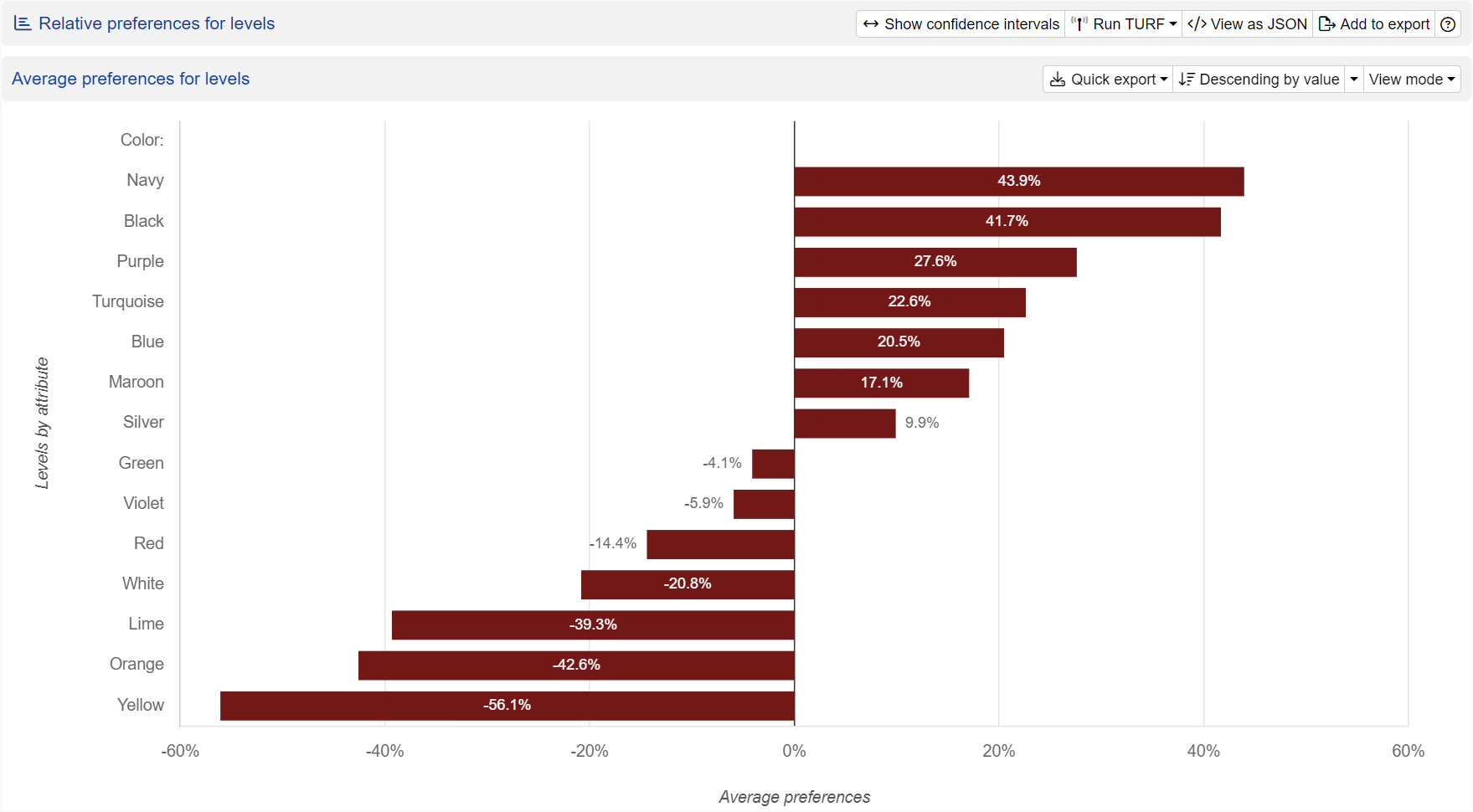
Relative value by levels
How do customers rank potential phone colour options?
Each level of each attribute is scored for its performance in customers’ decision-making. In our example, navy is the most favourable colour and is displayed as positive. Yellow is the least preferred colour and therefore displayed as negative. It's important to remember that the performance score of each attribute is relative to the other levels shown to respondents. For instance, the colour red will only be shown as negative when compared against a specific set of colours (levels) — testing red against a different range of colours could yield a positive result.
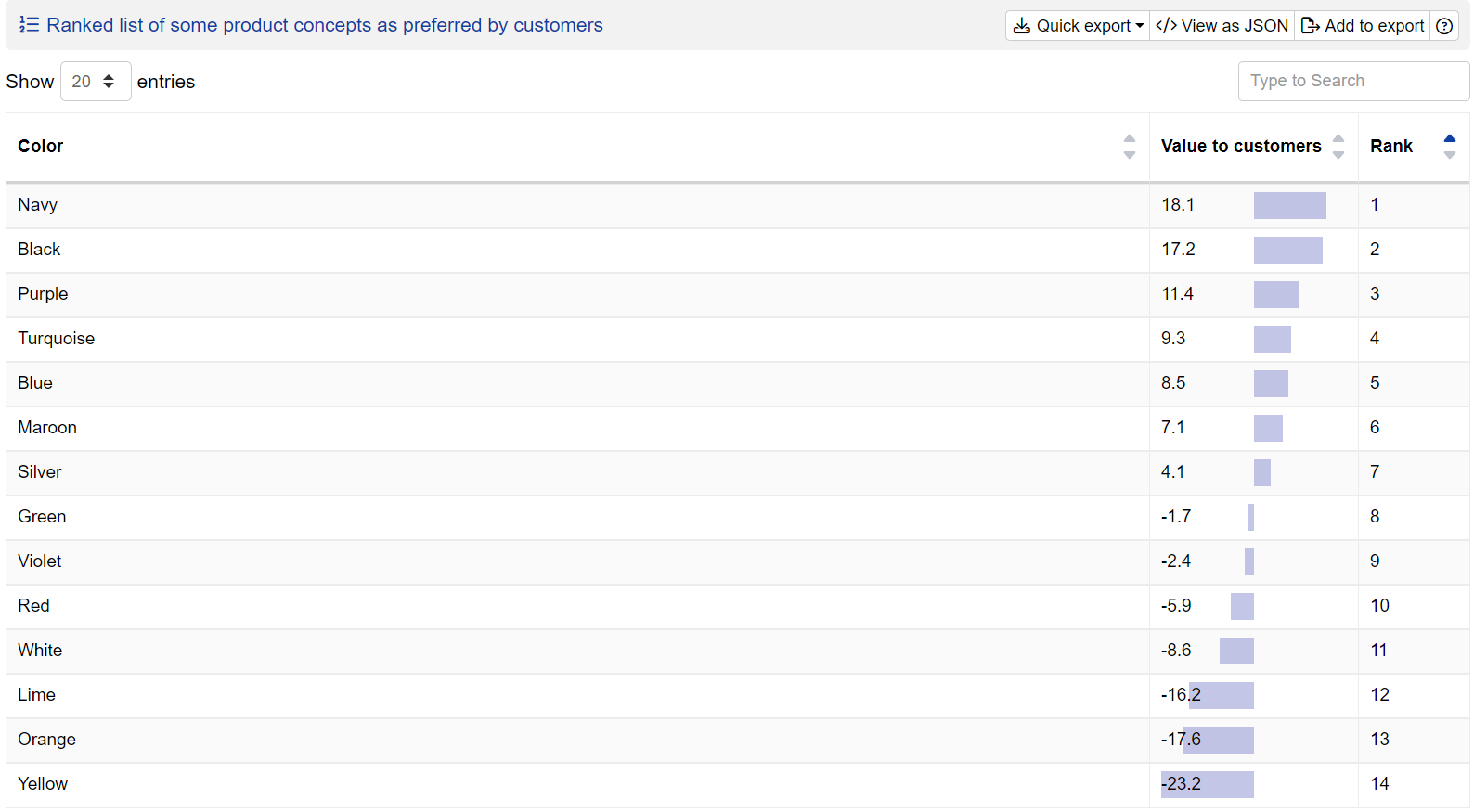
Ranked list of product constructs
List all possible level combinations and rank them by customers' preferences.
Conjointly forms the complete list of product constructs using all possible combinations of levels. They are then ranked based on the relative performance of the levels combined. This module allows you to find the best product construct that your customers will prefer over others.
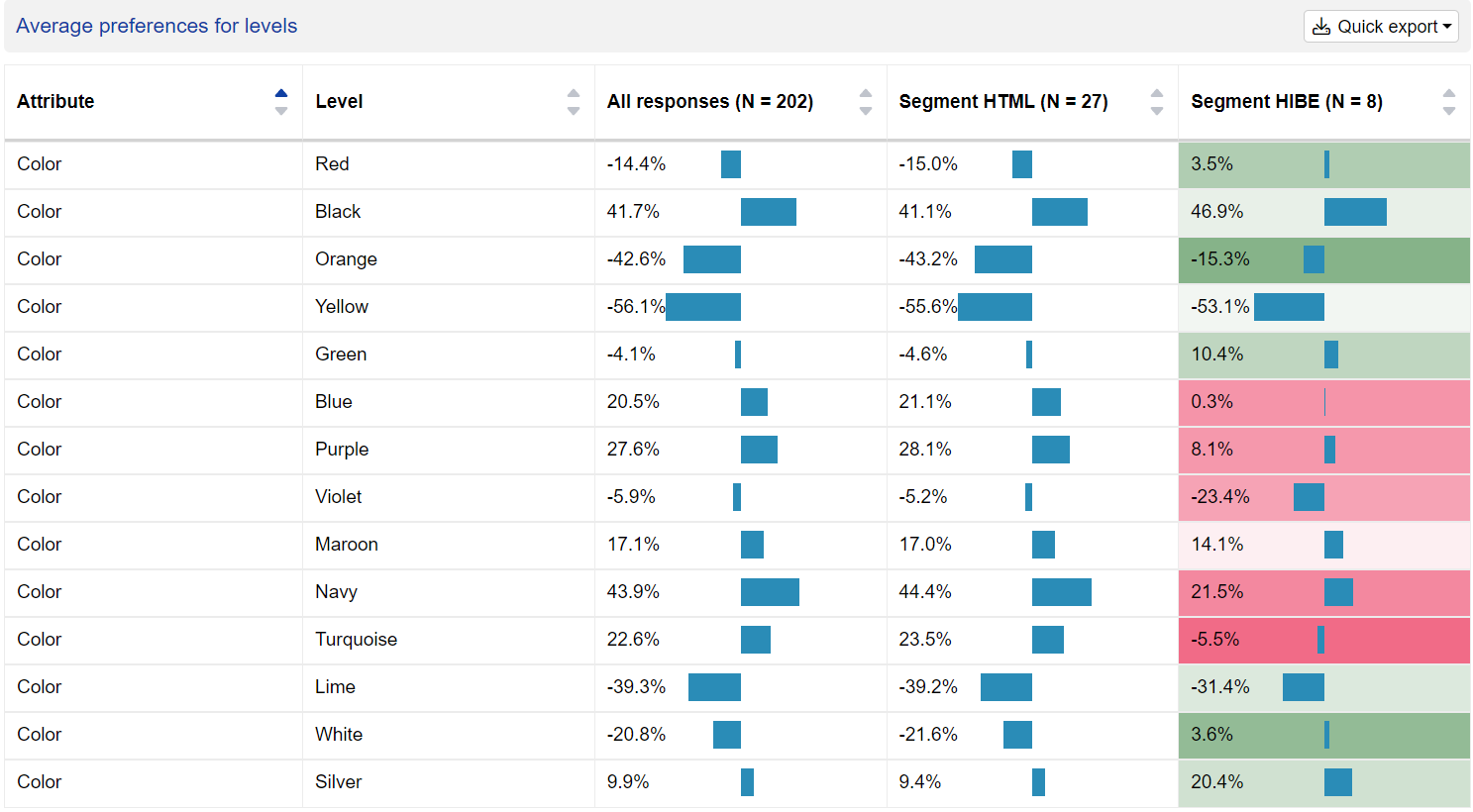
Segmentation of the market
Find out how preferences differ between segments.
With Conjointly, you can split your reports into various segments using the information collected automatically by our system, respondents' answers to additional questions (for example, multiple-choice and open-ends), GET variables, or sentiment analysis. For each segment, we provide the same detailed analytics as described above.
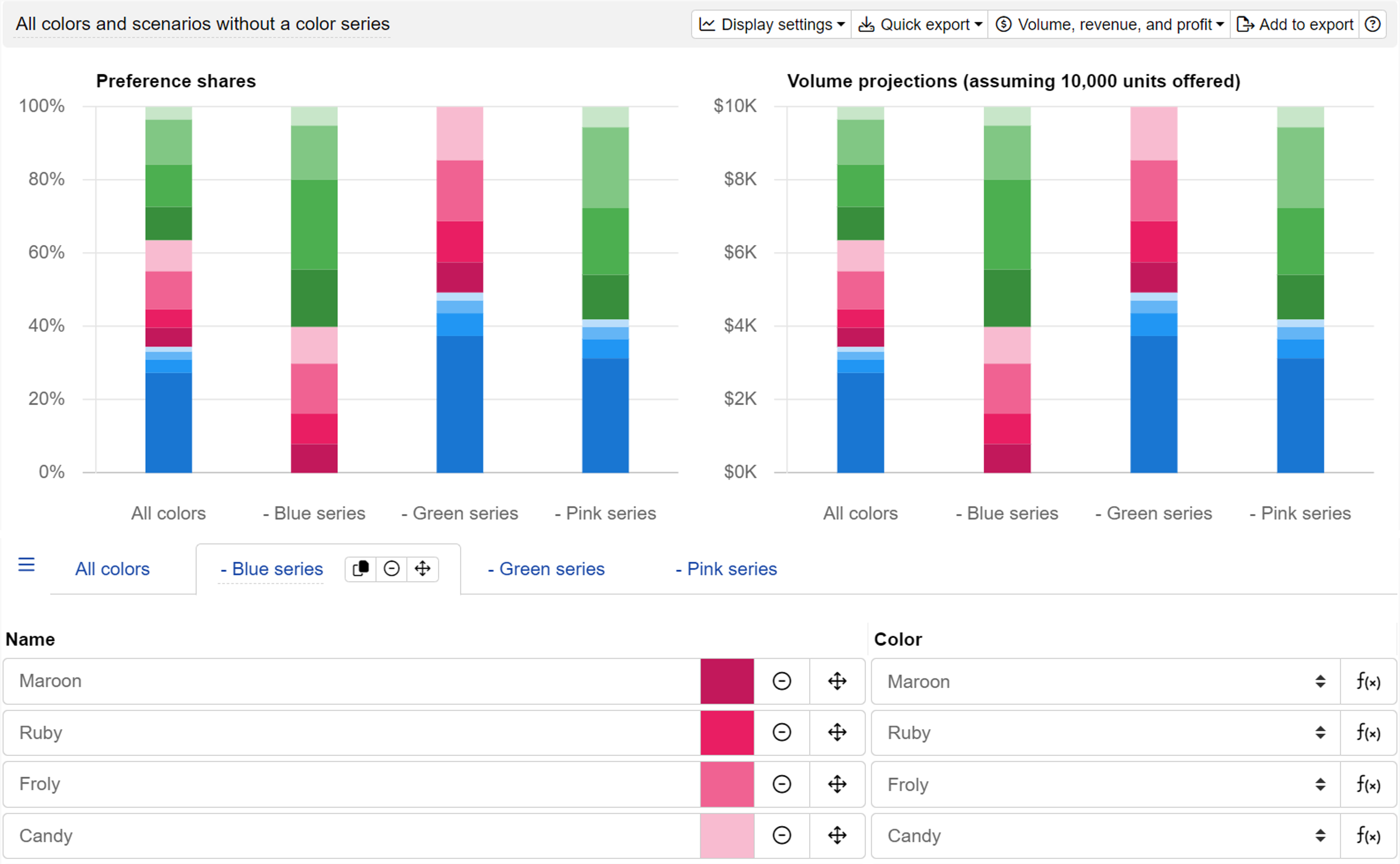
Preference share simulations
View simulations of preference shares for your product with the Preference Share Simulator.
With Conjointly, you can simulate shares of preference and volume projections for different product offerings, including those that are available in the market. Learn more about using the simulator for MaxDiff.
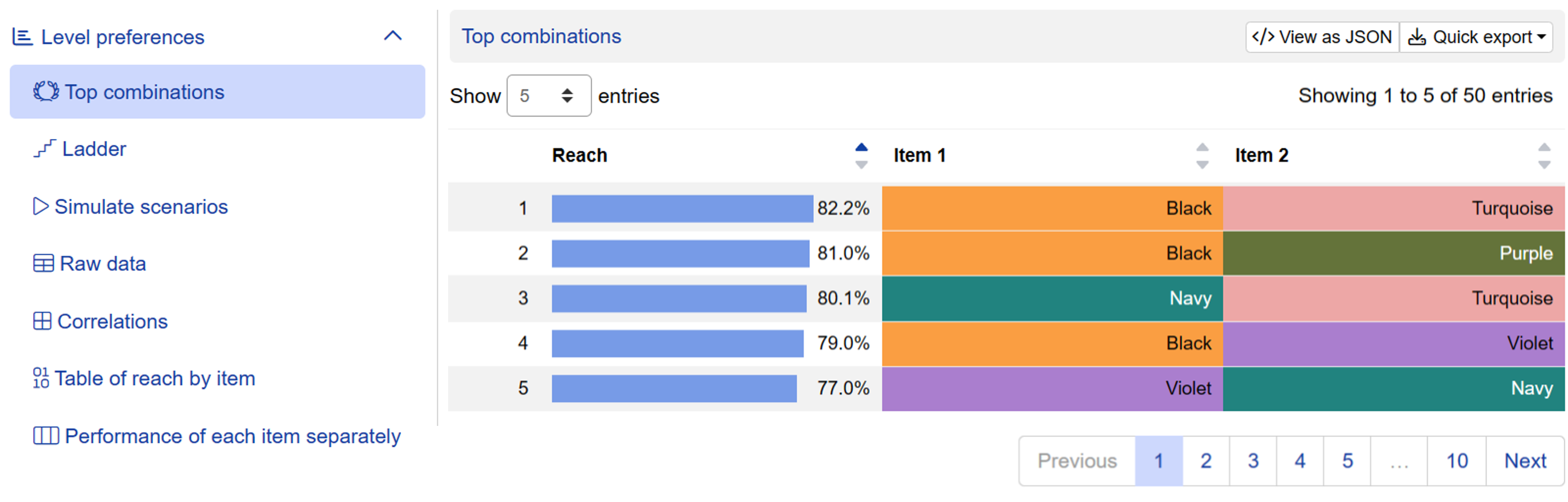
Analyse with TURF Simulator
Conduct TURF analysis on MaxDiff data using the TURF Analysis Simulator.
TURF analysis aims to find the combination of items that appeals to the largest proportion of consumers.
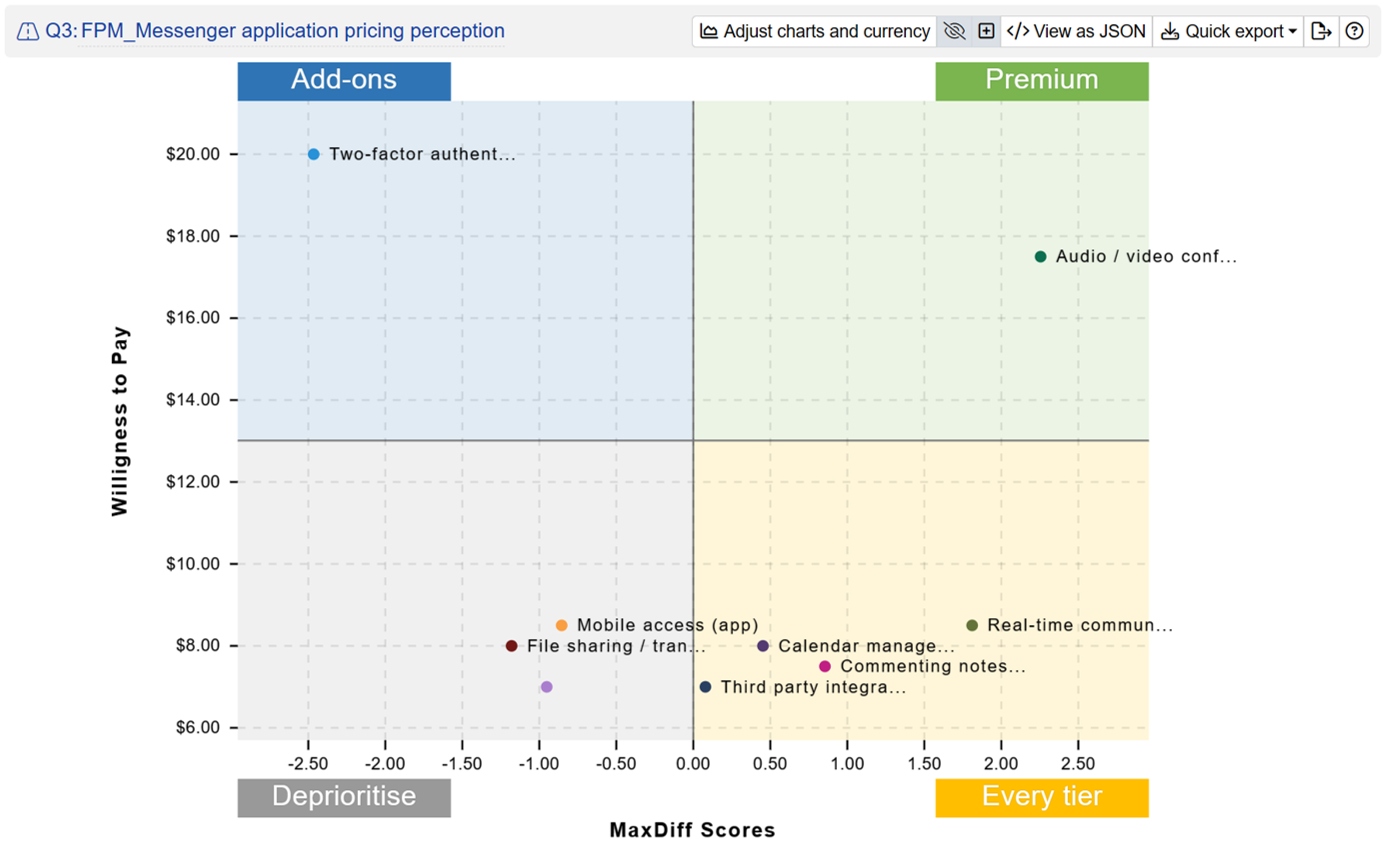
Feature Placement Matrix
Discover users' preferences and willingness to pay for each feature
A Feature Placement Matrix (FPM) is automatically generated for each Van Westendorp (VW) question included in your MaxDiff experiment when your sample size is 40 or more.
The matrix plots MaxDiff scores on the x-axis and the willingness to pay from the VW analysis on the y-axis, helping you classify product features into four quadrants:
- Premium: High feature importance & high willingness to pay.
- Add-ons: Low feature importance & high willingness to pay.
- Every tier: High feature importance & low willingness to pay.
- Deprioritise: Low feature importance & low willingness to pay.
Learn more about how the Feature Placement Matrix works.