What is Conjoint Analysis?
Conjoint analysis is a popular method of product and pricing research that uncovers consumers’ preferences, which is useful when a company wants to:
- Select product features.
- Assess consumers’ sensitivity to price changes.
- Forecast its volumes and market share.
- Predict adoption of new products or services.
Conjoint analysis is frequently used across different industries for all types of products, such as consumer goods, electrical goods, life insurance plans, retirement housing, luxury goods, and air travel. It is applicable in various instances that centre around discovering what type of product consumers are likely to buy and what consumers value the most (and least) about a product. As such, it is a familiar tool for marketers, product managers, and pricing specialists.
Businesses of all sizes can benefit from conjoint analysis, including even local grocery stores and restaurants — and its scope is not just limited to consumer contexts, for example, charities can use conjoint analysis’ techniques to find out donor preferences, while HR departments can use it to build optimal compensation packages.
How does conjoint analysis work?
Conjoint analysis works by breaking a product or service down into its components (attributes and levels) and testing different combinations of these components to identify consumer preferences.
For example, consider a conjoint study on smartphones. The smartphone is broken down into four attributes which are each assigned different possible variations to create levels:
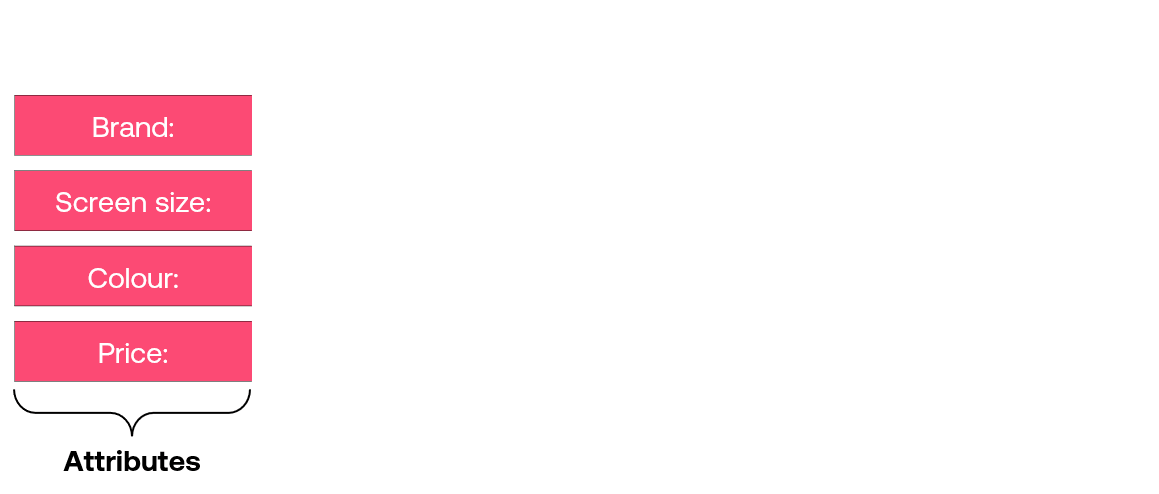
Each choice task then presents a respondent with different possible smartphones, each created by combining different levels for each attribute:
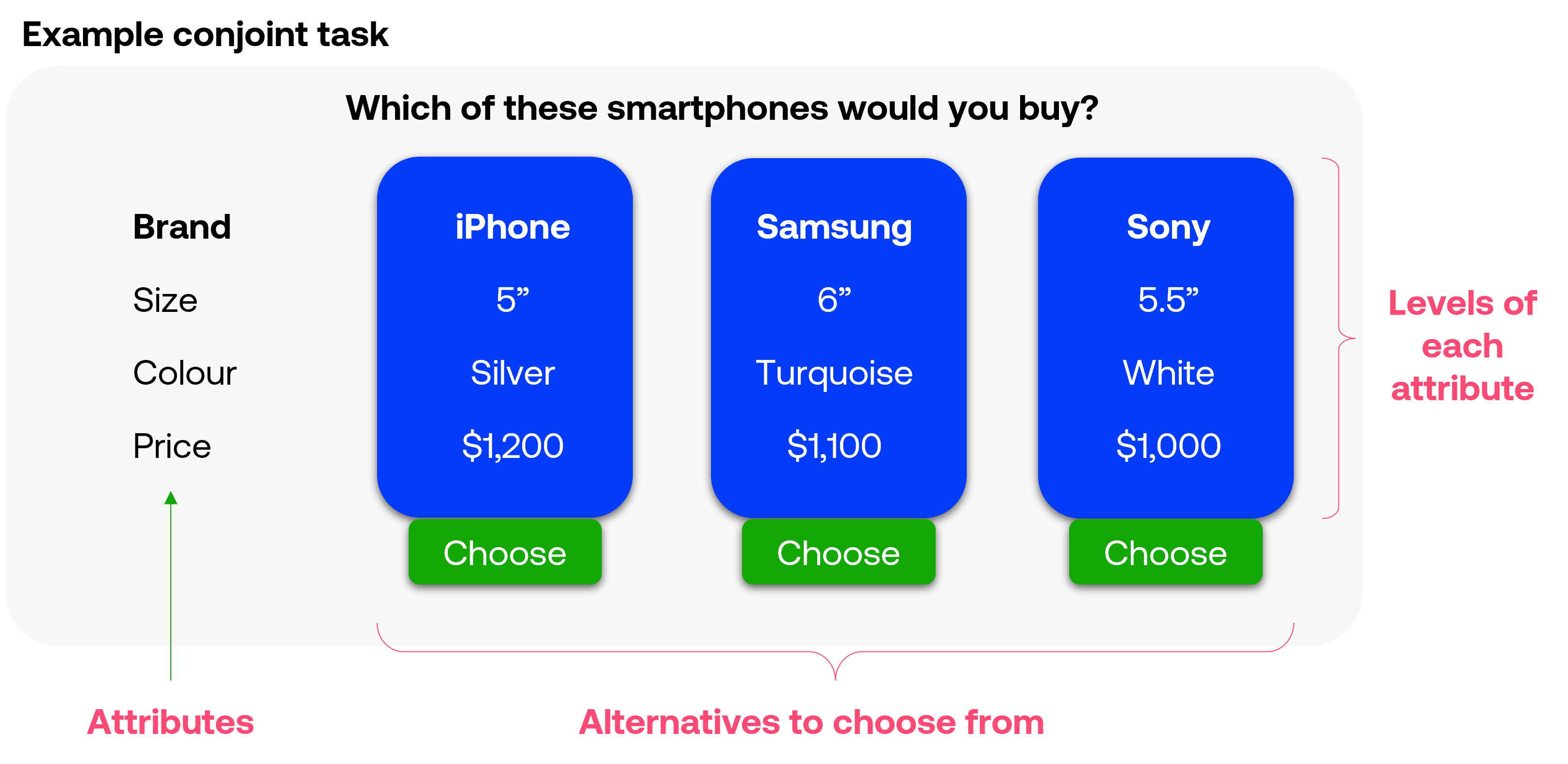
Going further than simply asking respondents what they like in a product, or what features they find most important, conjoint analysis employs a more realistic approach: asking each respondent to choose between potential product concepts (or alternatives) formed through the combination of attributes and levels. These combinations are carefully assembled into choice sets (or questions). Each respondent is typically presented with 8 to 12 questions. The process of assembling attributes and levels into product concepts and then into choice sets is called experimental design and requires extensive statistical and mathematical analysis (done automatically by Conjointly or manually by researchers).
Using survey results, it is possible to calculate a numerical value that measures how much each attribute and level influenced the respondent’s choices. Each of these values is called a “preference score” (AKA “partworth utility” or “utility score”). The below example shows preference scores for attributes and levels of a mobile phone plan.
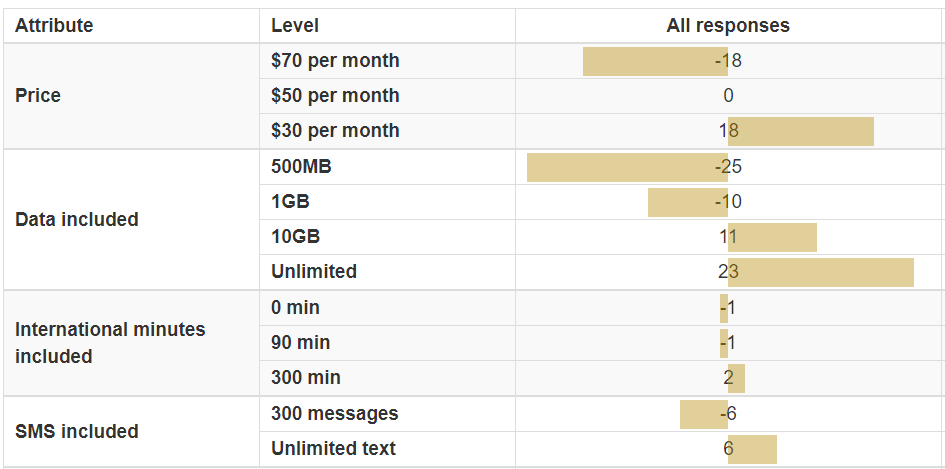
Preference scores are used to build simulators that forecast market shares for a set of different products offered to the market. By using the simulator to model (i.e. simulate) respondents’ decisions, we can identify the specific features and pricing that balance value to the customer with cost to the company and forecast potential demand in a competitive market situation. The below example shows how different data amounts in a mobile plan will affect a company’s market share.
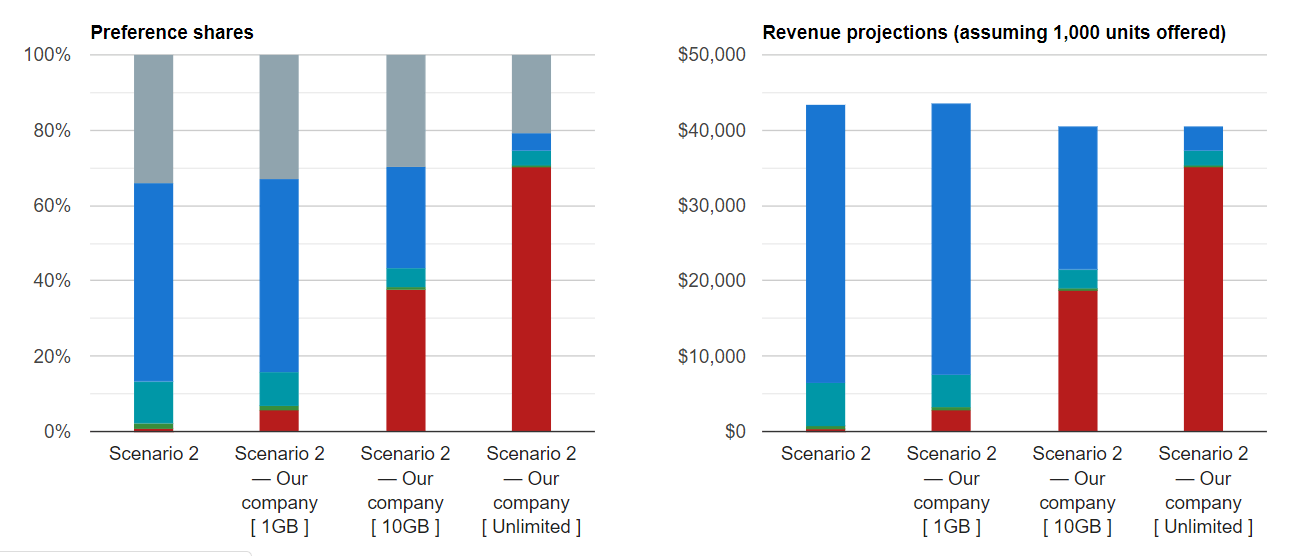
Outputs
Consider you are launching a new product and wish to address several research questions. Through the below example, we demonstrate how various outputs from your Conjointly survey report can be used to gain insights.
- To assess the relative importance of attributes (attribute preference scores/utilities, a.k.a. attribute partworth utilities). You can identify the most important attribute consumers look for when considering your product or service.
- To assess relative value by level (level preference scores/utilities, a.k.a. level partworth utilities). Consumer preferences are further broken down, displaying scores for each individual level.
- To evaluate adoption potential. You can simulate new product launches (NPDs) to see redistribution of preference and revenue shares.
- To test the impact of new features. Preference shares and revenue projections (assuming 1,000 units offered) can is simulated to assist in adding or modifying product features. In the below scenario a baseline for four car brands is being shown for preference shares and revenue projections.
- To find optimal pricing. Price elasticity of demand can be calculated for multiple scenarios by clicking on two points of a preference share simulation. In this scenario, when clicking on both the $23,000 and $25,000 price points for Ladina Klubnika a message will be displayed stating that the price elasticity of demand is elastic.
- To profile your potential customers. If you want to explore the characteristics of consumers who are most likely to purchase your product, you can segregate your sample based on preferences for concepts in a particular scenario.
- It is also possible to perform clustering based on raw conjoint utilities.
Why do conjoint analysis with Conjointly?
Conjointly automates the often complicated experimental design process using state-of-the-art methodology. This gives you control over specific settings, such as the number of concepts per choice set and the number of choice sets per respondent when you set up a conjoint analysis experiment. Respondents then complete the choice tasks within the conjoint survey – this typically requires a few hundred responses but may vary depending on the complexity of the study.
Once we’ve gathered the recommended sample size of respondents, Conjointly produces a survey report which contains several in-depth outputs. The outputs of Brand Specific Conjoint, Generic Conjoint, and Brand-Price Trade-Off include estimates of respondents’ preferences, overall sample profile, segmentation and interactive simulations. Conjointly estimates and charts preference shares, revenue projections, and price elasticity using simulators.
There are many types/flavours of conjoint analysis, classified by response type, questioning approach, design type, and adaptivity of the design. All flavours of conjoint analysis have the same basics but not all are as effective as others. That’s why Conjointly offers two key conjoint designs, called generic and brand-specific, and uses the most tested, developed, and theoretically sound response type – choice-based conjoint analysis (CBC). CBC’s predictive power far surpasses its alternatives, such as SIMALTO and self-explicated conjoint, making it the ideal choice for your next experiment.
Don’t have a large marketing budget or the scope to conduct conjoint analysis? That’s OK: Conjointly does full conjoint analysis for you, affordably. Unlike desktop software tools, Conjointly does not require you to deep dive into the advanced methodology of conjoint analysis. Your business can rely on the full functionality of the software to deliver high-quality analysis and powerfully accurate results. Conjointly embodies an agile approach that puts you in control of the research process without the need.
Conjointly is made unique by the following characteristics:
We are the home of conjoint analysis. Conjointly offers complete set of outputs and features through an accessible interface.
Quick to set up. Setting up your experiment is fast and hassle-free with a simple wizard, which helps you choose appropriate settings and suggests your minimum sample size. You won’t need to customise or test any survey – the system does that for you. Conjointly can send participants invites on your behalf or generate a shareable link for you.
Easy on respondents. Experiment participants only need a few minutes to complete a survey and can answer questions with ease on their mobile phone, tablet, or computer.
Smart analytics done for you. Behind the scenes, Conjointly uses state-of-the-art analytics to crunch the numbers, and check validity of reporting. Outputs are ready for any application of conjoint analysis (pricing, feature selection, product testing, new market entry, cannibalisation analysis, etc.) in any industry (telecommunications, SaaS, FMCG, automotive, financial services, HR, etc.).
Our market research experts are always ready to support your studies. Schedule a consultation if you need any assistance.
What is the difference between conjoint and discrete choice experiments?
Conjoint analysis is a survey-based quantitative research technique of presenting respondents with several options (each described in terms of feature and price levels) and measuring their response to these options.
When the measured response is their choice between these options (rather than ranking or rating each of these options), it is called choice-based conjoint (which is the most commonly-used type of discrete choice experiments).
Discrete choice analysis is examination of datasets that contain choices made by people from among several alternatives. Commonly, we want to understand what drove people to make these choices. For example, how does weather affect people’s choice of eating out, ordering food delivery, or cooking at home. Discrete choice analysis can be done on historical data (e.g. sales data) or from experiments (including survey-based experiments).
Choice-based conjoint is an example of discrete choice experimentation.
History of conjoint analysis
Conjoint analysis has its roots in academic research from the 1960s and has been used commercially since the 1970s. In 1964, two mathematicians, Duncan Luce and John Tukey published a rather indigestible (by modern standards) article called ‘Simultaneous conjoint measurement: A new type of fundamental measurement’. In abstract terms, they sketched the idea of “measuring the intrinsic goodness of certain characteristics of objects by measuring the goodness of an object as a whole”.
The article did not mention data collection, products, features, prices, or other elements that we associate with conjoint analysis today, but it spurred academic interest in the topic and perhaps gave rise to the name “conjoint”. It not only kick-started the topic but also set the tone for future developments in the area. Over time, it has become technical to the point of inaccessibility to most people, led by American academics with a strong emphasis on the statistical workings of survey research.
Green and Srinivasin (1978) agree that the theory of conjoint measurement was developed in Luce and Tukey’s paper but that “the first detailed, consumer-orientated” approach was Green and Rao’s (1971) ‘Conjoint Measurement for Quantifying Judgmental Data’. In 1974, Professor Paul E. Green penned ‘On the Design of Choice Experiments Involving Multifactor Alternatives’, cementing the impact of conjoint analysis in market research.
Over the next few decades, conjoint analysis became an increasingly popular method across the globe with notable studies in the 1980s and 90s highlighting its growing adoption and development during this time (Wittink & Cattin 1989; Wittink, Vriens, and Burhenne 1994 cited in Green, Kreiger & Wind 2001).
Conjoint surveys are continuously developing on a range of software platforms, through which many different flavours of conjoint analysis can be enjoyed. Today, conjoint analysis thrives as a widespread tool built on a robust methodology and is used by market researchers daily as an indispensable tool for understanding consumer trade-offs.
Example outputs of Generic Conjoint on ice-cream
This is a simple conjoint analysis report for a Generic Conjoint test on ice-cream. You can also take this survey yourself. We tested three features:
- Flavour (Fudge, Vanilla, Strawberry, and Mango)
- Size (from 120g to 200g)
- Price (from $1.95 to $3.50)
We collected over 1,500 good quality responses in this test (even though this report would be robust enough with a hundred complete answers). It turns out that variation of price was a more important driver of people’s decision-making than differences in both flavour and size of the cone combined:
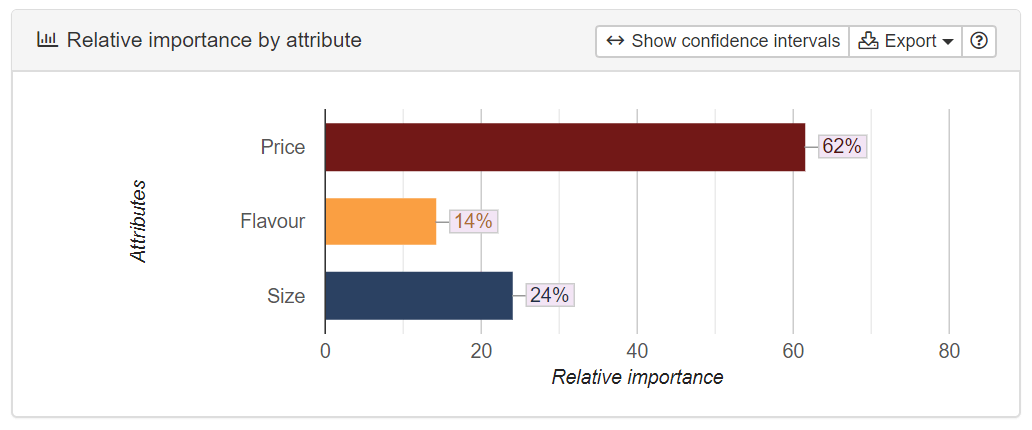
Unsurprisingly, people preferred larger and cheaper cones. Fudge and vanilla were the two top flavours:
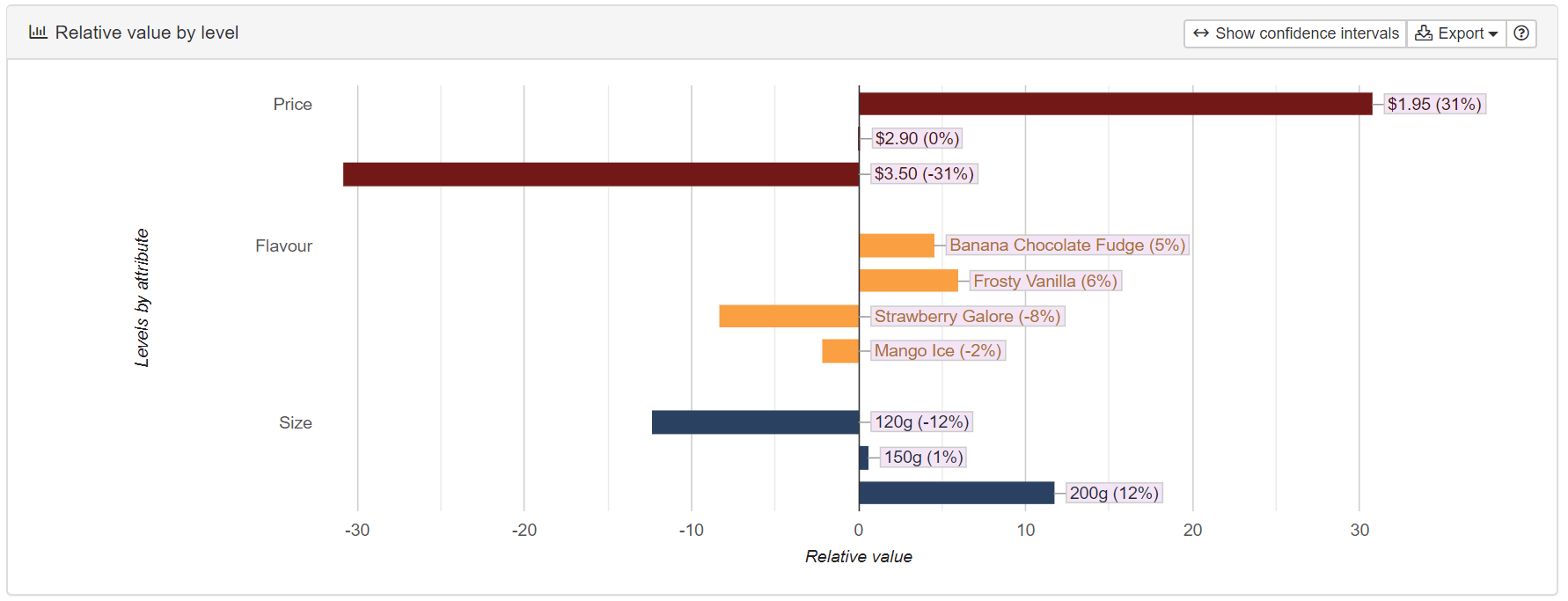
But when we look at confidence intervals, we notice that we are much less certain about average preferences for flavours than for size or price:
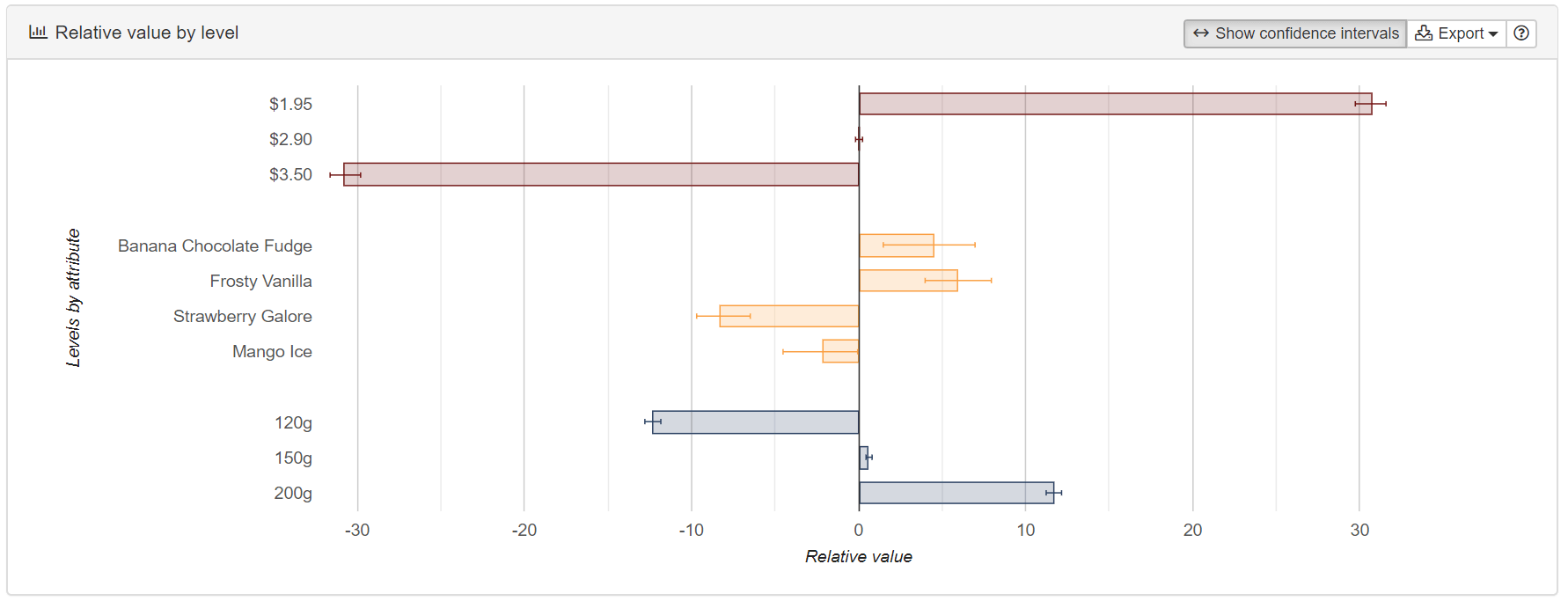
It is probably because if we simulate preference shares for four concepts with varied flavours but fixed price and size, we observe that the distribution of people who pick different options is not extremely skewed towards one flavour:
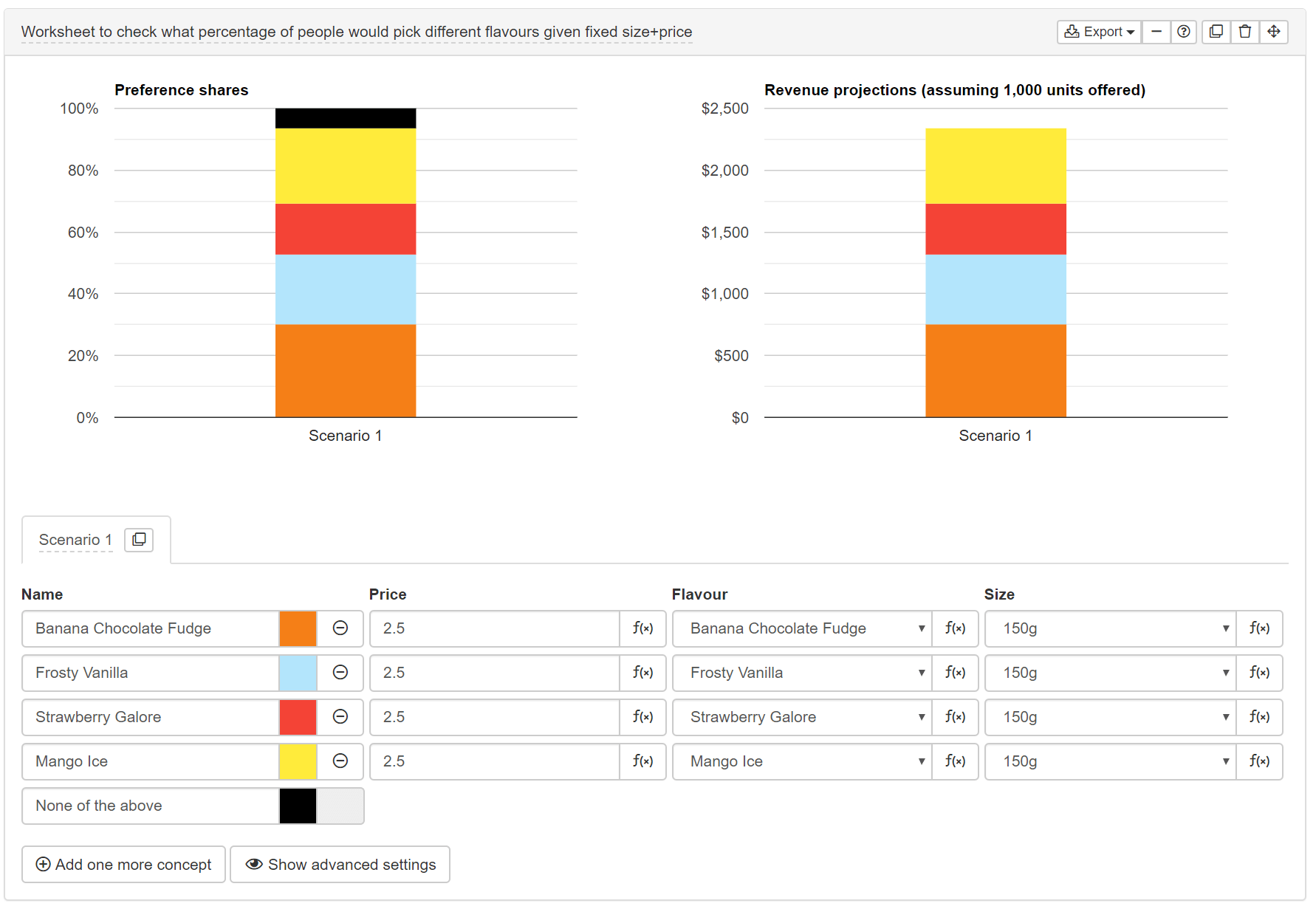
But when we do simulation analysis with different price points, we clearly see that more people prefer to pay a lower price. Even though some still stick with a higher price, probably due to price-quality inference.
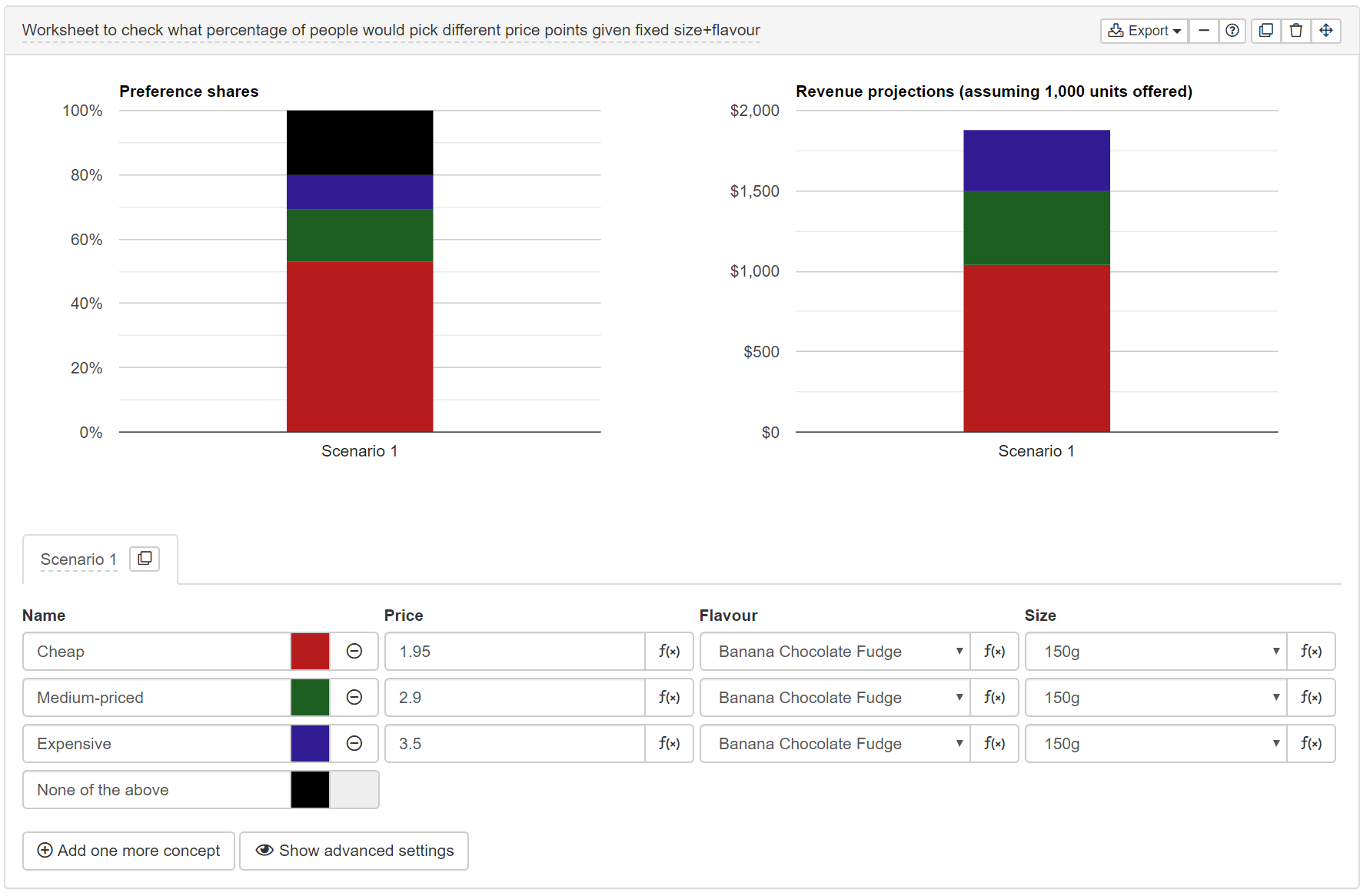
Another useful output of the study is marginal willingness to pay, which shows the equivalent amount of money for upgrade from the less preferred to the more preferred features:
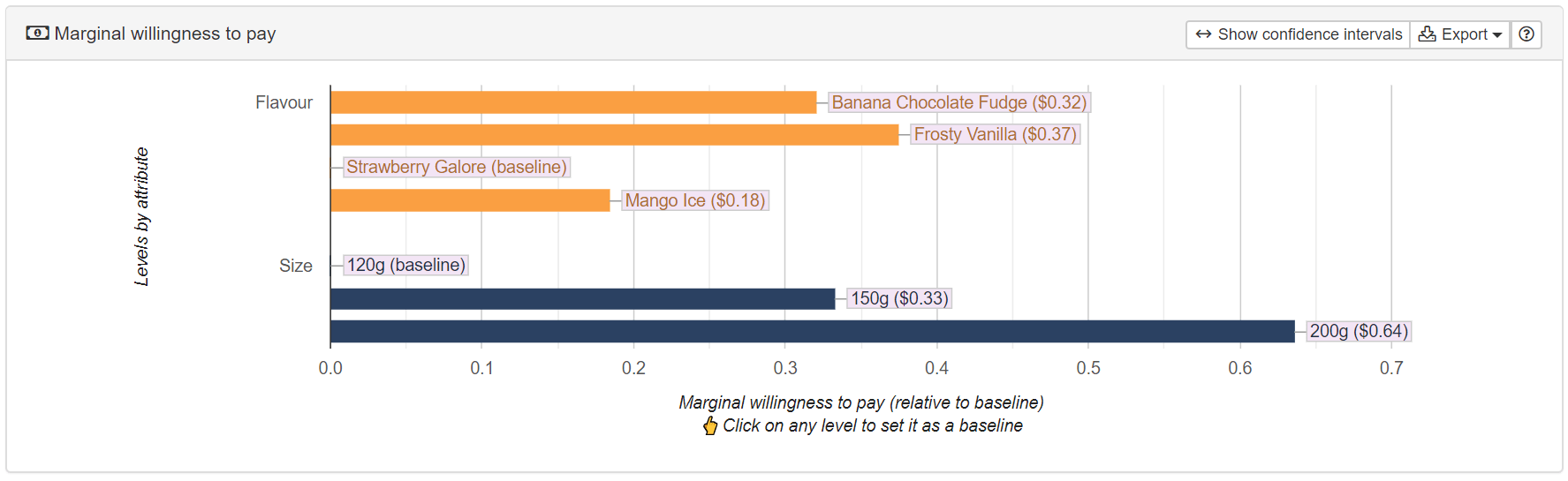
If you want to pick the topmost preferred combination of product features, you can take a look at the following ranking as well:
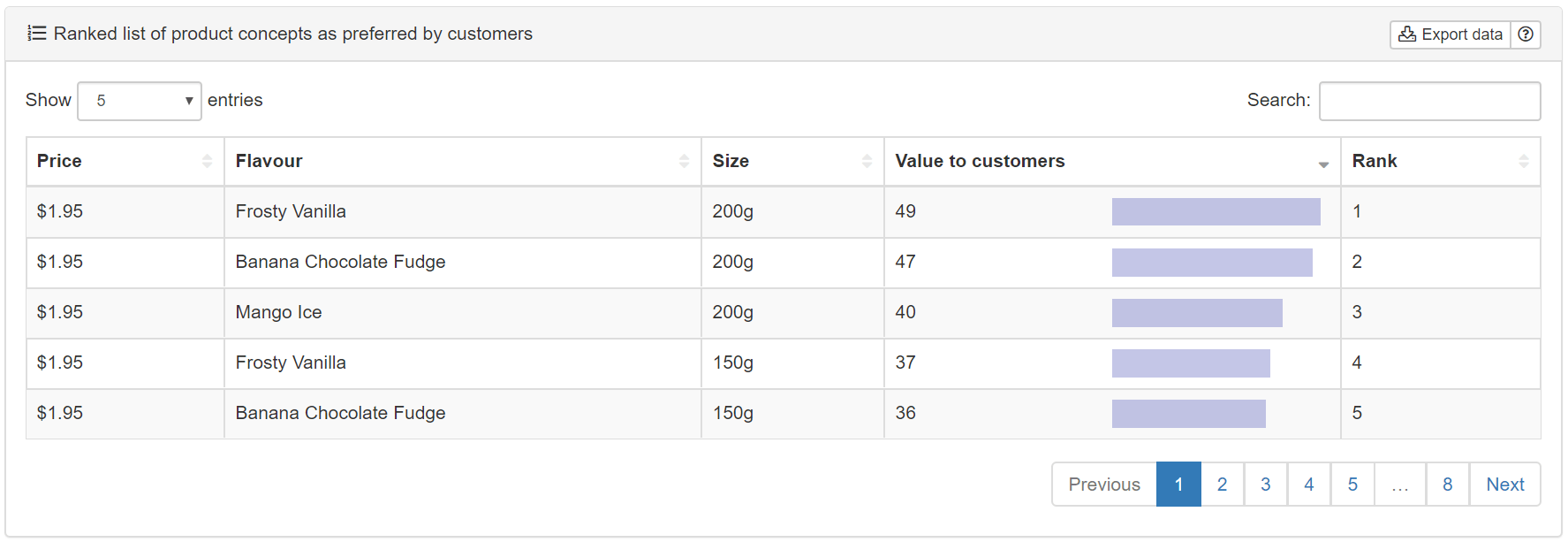
It looks like a large dollop of modestly-priced Frosty Vanilla is the winner today.
A simple conjoint analysis example in Excel
To further your understanding, you can download a conjoint analysis example in Excel, also available on Google Sheets (which you can copy to edit). This example covers:
- Inputs for a conjoint study
- Questions presented to respondents
- Calculations of preference scores (relative preferences and importance scores of attributes)
This example is limited to:
- Ten choice-based responses (in real conjoint tests, we collect ~12 choices from 100 to 2,000 respondents);
- Four attributes with two levels each (in real conjoint tests, we can have up to a dozen attributes and up to several dozen levels);
- A multiple linear regression (in real conjoint tests, we use hierarchical Bayesian multinomial logit);
- A fractional factorial design.
Next steps
The best way to learn more about conjoint analysis is to set up your own study, which you can do when you sign up. You can also read about:
- Classification of conjoint
- Alternatives to conjoint (such as MaxDiff and Claims Test)
- Common mistakes and practical tips for setting up conjoint studies
- Check out our webinar on Conjoint Analysis 101